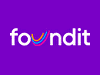
Computational Biologist (Microbiome Interaction Modelling), A*SRL
Computational Biologist (Microbiome Interaction Modelling)
We are seeking a highly motivated Computational Biologist to join the Host-Microbiota Ecology (HoME) lab at A* Skin Research Labs. The successful candidate will develop and implement computational models to study microbial interactions within the skin microbiome. This role will involve working closely with experimental scientists to integrate data from synthetic microbial communities and apply ecological theories and machine learning to predict microbiome dynamics. The role will be based at A*STAR Skin Research Labs on the Biopolis campus.
About the HoME Lab:
The Host-Microbiota Ecology (HoME) Lab focuses on understanding host-microbiota interactions through in vitro and in silico models, integrating synthetic ecology with computational modelling.
About the role
- Develop computational models (including ODE-based and agent-based models) to simulate microbial interactions.
- Analyze high-throughput data from synthetic microbiome experiments (e.g., transcriptomics and metabolomics).
- Collaborate with experimental biologists to design experiments that generate data for modelling.
- Implement machine learning algorithms for predicting microbial interactions and microbiome stability.
- Publish research findings in peer-reviewed journals and present at conferences.
Requirements
About the candidate
- PhD in Computational Biology, Bioinformatics, Systems Biology, or a related field.
Strong programming skills in MATLAB / Python / R or similar languages. - Experience with modelling biological systems using ODEs, agent-based models, or similar frameworks.
- Knowledge of microbiome research and microbial ecology is highly desirable. Familiarity with machine learning techniques and handling large biological datasets.
- Preferred candidate will have experience with microbiome data analysis and network modeling, background in ecology or systems biology, and experience in integrating experimental data with computational models.
Skills and abilities
- High level of organisational skills with the ability to plan and schedule multiple projects effectively.
- Ability to effectively handle both internal and external partners, some with competing agendas.
- Good verbal and written communication skills.
Personal Attributes
- Demonstrates initiative, creativity, flexibility and a personal ethos of continuous improvement.
- Flexible, professional and resourceful with a high level of organisational skills with the ability to prioritise, manage workload.
- Deliver agreed activities consistently on time.
- Ability to work independently when required.
Application instructions
Interested candidates should submit:
- A cover letter describing motivation and suitability for the role.
- CVComputational Biologist (Microbiome Interaction Modelling).
- A one-page writeup on their approach to one of their modelling work with a link to the publication, pre-print, or git-hub page.
- Contact information for three references.
Computational Biologist (Microbiome Interaction Modelling)
We are seeking a highly motivated Computational Biologist to join the Host-Microbiota Ecology (HoME) lab at A* Skin Research Labs. The successful candidate will develop and implement computational models to study microbial interactions within the skin microbiome. This role will involve working closely with experimental scientists to integrate data from synthetic microbial communities and apply ecological theories and machine learning to predict microbiome dynamics. The role will be based at A*STAR Skin Research Labs on the Biopolis campus.
About the HoME Lab:
The Host-Microbiota Ecology (HoME) Lab focuses on understanding host-microbiota interactions through in vitro and in silico models, integrating synthetic ecology with computational modelling.
About the role
- Develop computational models (including ODE-based and agent-based models) to simulate microbial interactions.
- Analyze high-throughput data from synthetic microbiome experiments (e.g., transcriptomics and metabolomics).
- Collaborate with experimental biologists to design experiments that generate data for modelling.
- Implement machine learning algorithms for predicting microbial interactions and microbiome stability.
- Publish research findings in peer-reviewed journals and present at conferences.
Requirements
About the candidate
- PhD in Computational Biology, Bioinformatics, Systems Biology, or a related field.
Strong programming skills in MATLAB / Python / R or similar languages. - Experience with modelling biological systems using ODEs, agent-based models, or similar frameworks.
- Knowledge of microbiome research and microbial ecology is highly desirable. Familiarity with machine learning techniques and handling large biological datasets.
- Preferred candidate will have experience with microbiome data analysis and network modeling, background in ecology or systems biology, and experience in integrating experimental data with computational models.
Skills and abilities
- High level of organisational skills with the ability to plan and schedule multiple projects effectively.
- Ability to effectively handle both internal and external partners, some with competing agendas.
- Good verbal and written communication skills.
Personal Attributes
- Demonstrates initiative, creativity, flexibility and a personal ethos of continuous improvement.
- Flexible, professional and resourceful with a high level of organisational skills with the ability to prioritise, manage workload.
- Deliver agreed activities consistently on time.
- Ability to work independently when required.
Application instructions
Interested candidates should submit:
- A cover letter describing motivation and suitability for the role.
- CVComputational Biologist (Microbiome Interaction Modelling).
- A one-page writeup on their approach to one of their modelling work with a link to the publication, pre-print, or git-hub page.
- Contact information for three references.